mirror of
https://github.com/nomic-ai/gpt4all.git
synced 2024-10-01 01:06:10 -04:00
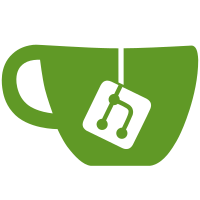
* new skeleton Signed-off-by: Max Cembalest <max@nomic.ai> * v3 docs Signed-off-by: Max Cembalest <max@nomic.ai> --------- Signed-off-by: Max Cembalest <max@nomic.ai>
177 lines
6.3 KiB
Markdown
177 lines
6.3 KiB
Markdown
# Embeddings
|
|
GPT4All supports generating high quality embeddings of arbitrary length text using any embedding model supported by llama.cpp.
|
|
|
|
An embedding is a vector representation of a piece of text. Embeddings are useful for tasks such as retrieval for
|
|
question answering (including retrieval augmented generation or *RAG*), semantic similarity search, classification, and
|
|
topic clustering.
|
|
|
|
## Supported Embedding Models
|
|
|
|
The following models have built-in support in Embed4All:
|
|
|
|
| Name | Embed4All `model_name` | Context Length | Embedding Length | File Size |
|
|
|--------------------|------------------------------------------------------|---------------:|-----------------:|----------:|
|
|
| [SBert] | all‑MiniLM‑L6‑v2.gguf2.f16.gguf | 512 | 384 | 44 MiB |
|
|
| [Nomic Embed v1] | nomic‑embed‑text‑v1.f16.gguf | 2048 | 768 | 262 MiB |
|
|
| [Nomic Embed v1.5] | nomic‑embed‑text‑v1.5.f16.gguf | 2048 | 64-768 | 262 MiB |
|
|
|
|
The context length is the maximum number of word pieces, or *tokens*, that a model can embed at once. Embedding texts
|
|
longer than a model's context length requires some kind of strategy; see [Embedding Longer Texts] for more information.
|
|
|
|
The embedding length is the size of the vector returned by `Embed4All.embed`.
|
|
|
|
[SBert]: https://huggingface.co/sentence-transformers/all-MiniLM-L6-v2
|
|
[Nomic Embed v1]: https://huggingface.co/nomic-ai/nomic-embed-text-v1
|
|
[Nomic Embed v1.5]: https://huggingface.co/nomic-ai/nomic-embed-text-v1.5
|
|
[Embedding Longer Texts]: #embedding-longer-texts
|
|
|
|
## Quickstart
|
|
```bash
|
|
pip install gpt4all
|
|
```
|
|
|
|
### Generating Embeddings
|
|
By default, embeddings will be generated on the CPU using all-MiniLM-L6-v2.
|
|
|
|
=== "Embed4All Example"
|
|
```py
|
|
from gpt4all import Embed4All
|
|
text = 'The quick brown fox jumps over the lazy dog'
|
|
embedder = Embed4All()
|
|
output = embedder.embed(text)
|
|
print(output)
|
|
```
|
|
=== "Output"
|
|
```
|
|
[0.034696947783231735, -0.07192722707986832, 0.06923297047615051, ...]
|
|
```
|
|
|
|
You can also use the GPU to accelerate the embedding model by specifying the `device` parameter. See the [GPT4All
|
|
constructor] for more information.
|
|
|
|
=== "GPU Example"
|
|
```py
|
|
from gpt4all import Embed4All
|
|
text = 'The quick brown fox jumps over the lazy dog'
|
|
embedder = Embed4All(device='gpu')
|
|
output = embedder.embed(text)
|
|
print(output)
|
|
```
|
|
=== "Output"
|
|
```
|
|
[0.034696947783231735, -0.07192722707986832, 0.06923297047615051, ...]
|
|
```
|
|
|
|
[GPT4All constructor]: gpt4all_python.md#gpt4all.gpt4all.GPT4All.__init__
|
|
|
|
### Nomic Embed
|
|
|
|
Embed4All has built-in support for Nomic's open-source embedding model, [Nomic Embed]. When using this model, you must
|
|
specify the task type using the `prefix` argument. This may be one of `search_query`, `search_document`,
|
|
`classification`, or `clustering`. For retrieval applications, you should prepend `search_document` for all of your
|
|
documents and `search_query` for your queries. See the [Nomic Embedding Guide] for more info.
|
|
|
|
=== "Nomic Embed Example"
|
|
```py
|
|
from gpt4all import Embed4All
|
|
text = 'Who is Laurens van der Maaten?'
|
|
embedder = Embed4All('nomic-embed-text-v1.f16.gguf')
|
|
output = embedder.embed(text, prefix='search_query')
|
|
print(output)
|
|
```
|
|
=== "Output"
|
|
```
|
|
[-0.013357644900679588, 0.027070969343185425, -0.0232995692640543, ...]
|
|
```
|
|
|
|
[Nomic Embed]: https://blog.nomic.ai/posts/nomic-embed-text-v1
|
|
[Nomic Embedding Guide]: https://docs.nomic.ai/atlas/guides/embeddings#embedding-task-types
|
|
|
|
### Embedding Longer Texts
|
|
|
|
Embed4All accepts a parameter called `long_text_mode`. This controls the behavior of Embed4All for texts longer than the
|
|
context length of the embedding model.
|
|
|
|
In the default mode of "mean", Embed4All will break long inputs into chunks and average their embeddings to compute the
|
|
final result.
|
|
|
|
To change this behavior, you can set the `long_text_mode` parameter to "truncate", which will truncate the input to the
|
|
sequence length of the model before generating a single embedding.
|
|
|
|
=== "Truncation Example"
|
|
```py
|
|
from gpt4all import Embed4All
|
|
text = 'The ' * 512 + 'The quick brown fox jumps over the lazy dog'
|
|
embedder = Embed4All()
|
|
output = embedder.embed(text, long_text_mode="mean")
|
|
print(output)
|
|
print()
|
|
output = embedder.embed(text, long_text_mode="truncate")
|
|
print(output)
|
|
```
|
|
=== "Output"
|
|
```
|
|
[0.0039850445464253426, 0.04558328539133072, 0.0035536508075892925, ...]
|
|
|
|
[-0.009771130047738552, 0.034792833030223846, -0.013273917138576508, ...]
|
|
```
|
|
|
|
|
|
### Batching
|
|
|
|
You can send multiple texts to Embed4All in a single call. This can give faster results when individual texts are
|
|
significantly smaller than `n_ctx` tokens. (`n_ctx` defaults to 2048.)
|
|
|
|
=== "Batching Example"
|
|
```py
|
|
from gpt4all import Embed4All
|
|
texts = ['The quick brown fox jumps over the lazy dog', 'Foo bar baz']
|
|
embedder = Embed4All()
|
|
output = embedder.embed(texts)
|
|
print(output[0])
|
|
print()
|
|
print(output[1])
|
|
```
|
|
=== "Output"
|
|
```
|
|
[0.03551332652568817, 0.06137588247656822, 0.05281158909201622, ...]
|
|
|
|
[-0.03879690542817116, 0.00013223080895841122, 0.023148687556385994, ...]
|
|
```
|
|
|
|
The number of texts that can be embedded in one pass of the model is proportional to the `n_ctx` parameter of Embed4All.
|
|
Increasing it may increase batched embedding throughput if you have a fast GPU, at the cost of VRAM.
|
|
```py
|
|
embedder = Embed4All(n_ctx=4096, device='gpu')
|
|
```
|
|
|
|
|
|
### Resizable Dimensionality
|
|
|
|
The embedding dimension of Nomic Embed v1.5 can be resized using the `dimensionality` parameter. This parameter supports
|
|
any value between 64 and 768.
|
|
|
|
Shorter embeddings use less storage, memory, and bandwidth with a small performance cost. See the [blog post] for more
|
|
info.
|
|
|
|
[blog post]: https://blog.nomic.ai/posts/nomic-embed-matryoshka
|
|
|
|
=== "Matryoshka Example"
|
|
```py
|
|
from gpt4all import Embed4All
|
|
text = 'The quick brown fox jumps over the lazy dog'
|
|
embedder = Embed4All('nomic-embed-text-v1.5.f16.gguf')
|
|
output = embedder.embed(text, dimensionality=64)
|
|
print(len(output))
|
|
print(output)
|
|
```
|
|
=== "Output"
|
|
```
|
|
64
|
|
[-0.03567073494195938, 0.1301717758178711, -0.4333043396472931, ...]
|
|
```
|
|
|
|
|
|
### API documentation
|
|
::: gpt4all.gpt4all.Embed4All
|