mirror of
https://github.com/oobabooga/text-generation-webui.git
synced 2024-10-01 01:26:03 -04:00
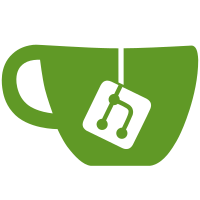
- Adds "still_streaming" to shared module for extensions to know if generation is complete - Changed TTS extension with new options: - Show text under the audio widget - Automatically play the audio once text generation finishes - manage the generated wav files (only keep files for finished generations, optional max file limit) - [wip] ability to change voice pitch and speed - added 'tensorboard' to requirements, since python sent "tensorboard not found" errors after a fresh installation.
213 lines
8.5 KiB
Python
213 lines
8.5 KiB
Python
import gc
|
|
import re
|
|
import time
|
|
|
|
import numpy as np
|
|
import torch
|
|
import transformers
|
|
from tqdm import tqdm
|
|
|
|
import modules.shared as shared
|
|
from modules.extensions import apply_extensions
|
|
from modules.html_generator import generate_4chan_html, generate_basic_html
|
|
from modules.models import local_rank
|
|
from modules.stopping_criteria import _SentinelTokenStoppingCriteria
|
|
|
|
|
|
def get_max_prompt_length(tokens):
|
|
max_length = 2048-tokens
|
|
if shared.soft_prompt:
|
|
max_length -= shared.soft_prompt_tensor.shape[1]
|
|
return max_length
|
|
|
|
def encode(prompt, tokens_to_generate=0, add_special_tokens=True):
|
|
|
|
# These models do not have explicit tokenizers for now, so
|
|
# we return an estimate for the number of tokens
|
|
if shared.is_RWKV or shared.is_LLaMA:
|
|
return np.zeros((1, len(prompt)//4))
|
|
|
|
input_ids = shared.tokenizer.encode(str(prompt), return_tensors='pt', truncation=True, max_length=get_max_prompt_length(tokens_to_generate), add_special_tokens=add_special_tokens)
|
|
if shared.args.cpu:
|
|
return input_ids
|
|
elif shared.args.flexgen:
|
|
return input_ids.numpy()
|
|
elif shared.args.deepspeed:
|
|
return input_ids.to(device=local_rank)
|
|
else:
|
|
return input_ids.cuda()
|
|
|
|
def decode(output_ids):
|
|
reply = shared.tokenizer.decode(output_ids, skip_special_tokens=True)
|
|
reply = reply.replace(r'<|endoftext|>', '')
|
|
return reply
|
|
|
|
def generate_softprompt_input_tensors(input_ids):
|
|
inputs_embeds = shared.model.transformer.wte(input_ids)
|
|
inputs_embeds = torch.cat((shared.soft_prompt_tensor, inputs_embeds), dim=1)
|
|
filler_input_ids = torch.zeros((1, inputs_embeds.shape[1]), dtype=input_ids.dtype).to(shared.model.device)
|
|
#filler_input_ids += shared.model.config.bos_token_id # setting dummy input_ids to bos tokens
|
|
return inputs_embeds, filler_input_ids
|
|
|
|
# Removes empty replies from gpt4chan outputs
|
|
def fix_gpt4chan(s):
|
|
for i in range(10):
|
|
s = re.sub("--- [0-9]*\n>>[0-9]*\n---", "---", s)
|
|
s = re.sub("--- [0-9]*\n *\n---", "---", s)
|
|
s = re.sub("--- [0-9]*\n\n\n---", "---", s)
|
|
return s
|
|
|
|
# Fix the LaTeX equations in galactica
|
|
def fix_galactica(s):
|
|
s = s.replace(r'\[', r'$')
|
|
s = s.replace(r'\]', r'$')
|
|
s = s.replace(r'\(', r'$')
|
|
s = s.replace(r'\)', r'$')
|
|
s = s.replace(r'$$', r'$')
|
|
s = re.sub(r'\n', r'\n\n', s)
|
|
s = re.sub(r"\n{3,}", "\n\n", s)
|
|
return s
|
|
|
|
def formatted_outputs(reply, model_name):
|
|
if not (shared.args.chat or shared.args.cai_chat):
|
|
if model_name.lower().startswith('galactica'):
|
|
reply = fix_galactica(reply)
|
|
return reply, reply, generate_basic_html(reply)
|
|
elif model_name.lower().startswith(('gpt4chan', 'gpt-4chan', '4chan')):
|
|
reply = fix_gpt4chan(reply)
|
|
return reply, 'Only applicable for GALACTICA models.', generate_4chan_html(reply)
|
|
else:
|
|
return reply, 'Only applicable for GALACTICA models.', generate_basic_html(reply)
|
|
else:
|
|
return reply
|
|
|
|
def generate_reply(question, max_new_tokens, do_sample, temperature, top_p, typical_p, repetition_penalty, top_k, min_length, no_repeat_ngram_size, num_beams, penalty_alpha, length_penalty, early_stopping, eos_token=None, stopping_string=None):
|
|
gc.collect()
|
|
if not shared.args.cpu:
|
|
torch.cuda.empty_cache()
|
|
|
|
t0 = time.time()
|
|
|
|
# These models are not part of Hugging Face, so we handle them
|
|
# separately and terminate the function call earlier
|
|
if shared.is_RWKV or shared.is_LLaMA:
|
|
if shared.args.no_stream:
|
|
reply = shared.model.generate(question, token_count=max_new_tokens, temperature=temperature, top_p=top_p)
|
|
t1 = time.time()
|
|
print(f"Output generated in {(t1-t0):.2f} seconds.")
|
|
yield formatted_outputs(reply, shared.model_name)
|
|
else:
|
|
for i in tqdm(range(max_new_tokens//8+1)):
|
|
reply = shared.model.generate(question, token_count=8, temperature=temperature, top_p=top_p)
|
|
yield formatted_outputs(reply, shared.model_name)
|
|
question = reply
|
|
return
|
|
|
|
original_question = question
|
|
if not (shared.args.chat or shared.args.cai_chat):
|
|
question = apply_extensions(question, "input")
|
|
if shared.args.verbose:
|
|
print(f"\n\n{question}\n--------------------\n")
|
|
|
|
input_ids = encode(question, max_new_tokens)
|
|
cuda = "" if (shared.args.cpu or shared.args.deepspeed or shared.args.flexgen) else ".cuda()"
|
|
n = shared.tokenizer.eos_token_id if eos_token is None else encode(eos_token)[0][-1]
|
|
|
|
if stopping_string is not None:
|
|
# The stopping_criteria code below was copied from
|
|
# https://github.com/PygmalionAI/gradio-ui/blob/master/src/model.py
|
|
t = encode(stopping_string, 0, add_special_tokens=False)
|
|
stopping_criteria_list = transformers.StoppingCriteriaList([
|
|
_SentinelTokenStoppingCriteria(
|
|
sentinel_token_ids=t,
|
|
starting_idx=len(input_ids[0])
|
|
)
|
|
])
|
|
else:
|
|
stopping_criteria_list = None
|
|
|
|
if not shared.args.flexgen:
|
|
generate_params = [
|
|
f"eos_token_id={n}",
|
|
f"stopping_criteria=stopping_criteria_list",
|
|
f"do_sample={do_sample}",
|
|
f"temperature={temperature}",
|
|
f"top_p={top_p}",
|
|
f"typical_p={typical_p}",
|
|
f"repetition_penalty={repetition_penalty}",
|
|
f"top_k={top_k}",
|
|
f"min_length={min_length if shared.args.no_stream else 0}",
|
|
f"no_repeat_ngram_size={no_repeat_ngram_size}",
|
|
f"num_beams={num_beams}",
|
|
f"penalty_alpha={penalty_alpha}",
|
|
f"length_penalty={length_penalty}",
|
|
f"early_stopping={early_stopping}",
|
|
]
|
|
else:
|
|
generate_params = [
|
|
f"do_sample={do_sample}",
|
|
f"temperature={temperature}",
|
|
f"stop={n}",
|
|
]
|
|
|
|
if shared.args.deepspeed:
|
|
generate_params.append("synced_gpus=True")
|
|
if shared.args.no_stream:
|
|
generate_params.append("max_new_tokens=max_new_tokens")
|
|
else:
|
|
generate_params.append("max_new_tokens=8")
|
|
|
|
if shared.soft_prompt:
|
|
inputs_embeds, filler_input_ids = generate_softprompt_input_tensors(input_ids)
|
|
generate_params.insert(0, "inputs_embeds=inputs_embeds")
|
|
generate_params.insert(0, "filler_input_ids")
|
|
else:
|
|
generate_params.insert(0, "input_ids")
|
|
|
|
# Generate the entire reply at once
|
|
if shared.args.no_stream:
|
|
with torch.no_grad():
|
|
output = eval(f"shared.model.generate({', '.join(generate_params)}){cuda}")[0]
|
|
if shared.soft_prompt:
|
|
output = torch.cat((input_ids[0], output[filler_input_ids.shape[1]:]))
|
|
|
|
reply = decode(output)
|
|
if not (shared.args.chat or shared.args.cai_chat):
|
|
reply = original_question + apply_extensions(reply[len(question):], "output")
|
|
|
|
t1 = time.time()
|
|
print(f"Output generated in {(t1-t0):.2f} seconds ({(len(output)-len(input_ids[0]))/(t1-t0)/8:.2f} it/s, {len(output)-len(input_ids[0])} tokens)")
|
|
yield formatted_outputs(reply, shared.model_name)
|
|
|
|
# Generate the reply 8 tokens at a time
|
|
else:
|
|
yield formatted_outputs(original_question, shared.model_name)
|
|
shared.still_streaming = True
|
|
for i in tqdm(range(max_new_tokens//8+1)):
|
|
with torch.no_grad():
|
|
output = eval(f"shared.model.generate({', '.join(generate_params)}){cuda}")[0]
|
|
if shared.soft_prompt:
|
|
output = torch.cat((input_ids[0], output[filler_input_ids.shape[1]:]))
|
|
|
|
reply = decode(output)
|
|
if not (shared.args.chat or shared.args.cai_chat):
|
|
reply = original_question + apply_extensions(reply[len(question):], "output")
|
|
|
|
if not shared.args.flexgen:
|
|
if output[-1] == n:
|
|
break
|
|
input_ids = torch.reshape(output, (1, output.shape[0]))
|
|
else:
|
|
if np.count_nonzero(input_ids[0] == n) < np.count_nonzero(output == n):
|
|
break
|
|
input_ids = np.reshape(output, (1, output.shape[0]))
|
|
|
|
#Mid-stream yield, ran if no breaks
|
|
yield formatted_outputs(reply, shared.model_name)
|
|
|
|
if shared.soft_prompt:
|
|
inputs_embeds, filler_input_ids = generate_softprompt_input_tensors(input_ids)
|
|
|
|
#Stream finished from max tokens or break. Do final yield.
|
|
shared.still_streaming = False
|
|
yield formatted_outputs(reply, shared.model_name) |